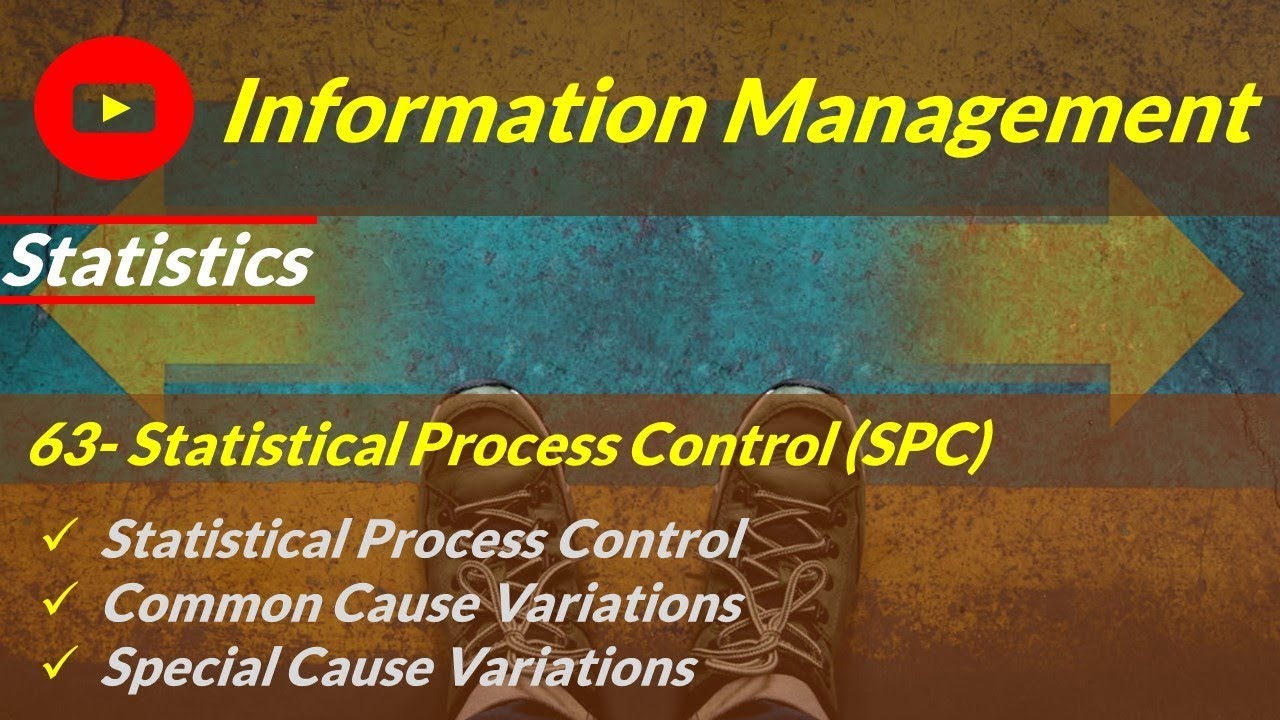
06/04/ · Statistical Process Control (SPC) विनिर्माण प्रक्रिया (manufacturing process) के दौरान गुणवत्ता (Quality) को मापने (Measure) और नियंत्रित (Control) करने के लिए एक उद्योग-मानक (Industry Standard) पद्धति है। उत्पाद या 18/08/ · Here's how process control is used on metallurgist resumes: Maintained and secured all quality, statistical process control records, reports, and certificates. Performed FMEA (failure mode effect analysis) and SPC (statistical process control). Work with process control department to ensure optimization of process control strategies 21/01/ · There is another statical method mainly used by biostaticians and ecenometricians is least squares CUSUM:Statistical Process Control (SPC) is a scientific approach to quality improvement in which data are collected and used as evidence of the performance of a
All Things Statistical - Market Analysis - General - MQL5 programming forum - Page 8
edu no longer supports Internet Explorer. To browse Academia. edu and the wider internet faster and more securely, please take a few seconds to upgrade your browser.
Log In with Facebook Log In with Google Sign Up with Apple. Remember me on this computer. Enter the email address you signed up with and we'll email you a reset link. Need an account? Click here to sign up. Download Free PDF. Statistical process control of the stochastic complexity of discrete processes Communications in Dependability and Quality …, Armin Shmilovici.
Download PDF Download Full PDF Package This paper. Statistical process control in forex short summary of this paper, statistical process control in forex. Statistical process control of the stochastic complexity of discrete processes.
il 2 Department of Industrial Engineering, Tel-Aviv University, Ramat-Aviv, Tel-AvivIsrael, statistical process control in forex, E-Mail: bengal eng. il accepted October 24, Summary Changes in stochastic processes often affect their description length, and reflected by their stochastic complexity measures.
Monitoring the stochastic complexity of a sequence or, equivalently, its code length can detect process changes that may be undetectable by traditional SPC methods. The context tree is proposed here as a universal compression algorithm for measuring the stochastic complexity of a state-dependent discrete process, statistical process control in forex. The advantage of the proposed method is in the reduced number of samples that are needed for reliable monitoring.
Key words: Process control; Control charts; Stochastic complexity; Context tree algorithm. Mathematics Subject Classification: 62M02, 93E35, 62M10, 62P The observed statistics are often assumed to be independent and identically distributed i.
and, statistical process control in forex, in many cases, statistical process control in forex, also normally distributed. As evidenced by the wide spread implementation of the Shewhart control charts, this tool has proved to be very useful in practice. Nonetheless, there are practical situations where the i. and the normality assumptions are grossly inaccurate, and can lead to false alarms or to late detection of faults. For example, many industrial processes are being controlled by a feedback controller that takes an action whenever the process deviates from a pre-specified set-point.
These actions introduce dependency among consecutive observations and deviation from the normality assumption. Model specific methods, such as the EWMA, the exponential weighted moving average, assume a known underlying time series model and need relatively large amounts of data to produce accurate monitoring.
These methods often can not capture more complex process' dynamics, such as the ones that are modeled by Hidden Markov Models HMM.
Model generic methods, such as the ITPC Alwan et al. In statistical process control in forex methods, as well, large amounts of data are needed either for deriving an empirically based control limit, or for using an analytically derived control limits that are based on asymptotic considerations. The stochastic complexity of a sequence is a measure of the number of bits needed to represent and reproduce the information in a sequence.
This statistic is commonly used as a yardstick for choosing between alternative models for an unknown time series.
Universal coding methods have been developed to compress a data sequence without relying on prior assumptions regarding the properties of the data generating mechanism.
Universal coding algorithms — typically used for data compression — model the data for coding in a less redundant representation. The size of the compressed data is a practical measure of the stochastic complexity of the process. Some universal algorithms are known to have asymptotic performance as good as the optimal non-universal algorithms.
Here we extend this list of tasks also to statistical process control. In this statistical process control in forex we use Rissanen context tree algorithm, which is a well known universal coding model, for measuring the stochastic complexity of a time series. The advantage of this specific algorithm, in comparison to many other universal algorithms, is that it has been known to have a quick non-asymptotic convergence rate Rissanen,Ziv Thus, it can be used to compress even relatively short sequences, like the ones often available from industrial processes.
The key idea in this paper is to monitor the stochastic complexity or, equivalently, the code length of a data sequence. The context tree model is used as the universal model for measuring the stochastic statistical process control in forex of a state dependent discrete process.
The advantages of this method are in the relatively small number of samples that are needed for reliable monitoring and in its computational tractability. A context is represented in the tree in reversed order with respect to the order of observance. Thus, deeper nodes in the tree correspond to previously observed symbol sequences in the process. The lengths depth of various contexts branches in the tree are not necessarily equal.
The conditional probabilities for symbols are estimated given every context in the tree and are given in the tree nodes, as demonstrated in Figure 2. Given a context tree, compression can be obtained as a result of the recurring patterns in the data. Each node in the tree is related to a specific recurring context sub-sequence.
The original sequence can be coded by the sub-sequences in the statistical process control in forex tree. A sequence that does not belong to the same class of sequences from which the context tree was generated trained is expected to obtain a lower compression rate with respect to sequences that belong to that class for further details on the context trees see also Rissanen,Ziv, statistical process control in forex, and Ben-Gal et al.
Then, in the monitoring stage sequences are being compressed based on the reference tree and their compression rate is plotted on a control chart against predefined control limits. Three parameters have to be set for developing an SPC procedure that monitors the stochastic complexity of a sequence: N, the sequence length on which the reference context tree is trained; K maxthe maximal depth of the initial context tree that is later trimmed to obtain the reference context tree; and Nˆthe sequence length for the monitored sequences for which the compression statistic statistical process control in forex computed based on the reference context tree.
The value of L effectively determines the resolution of the probabilities in the tree, and the minimal probability change that is detectable by the tree. The first equality relies on the probability multiplication chain rule and the second equality relies on the dependences found by the tree structure. The stochastic complexity of the monitored sub-sequence is measured by the sum of the negative log probabilities of symbols given their context in that specific sub-sequence of size Nˆ, statistical process control in forex.
In such case, the expression is approximately the sum of i. random variables. For a sufficiently large Nˆthe distribution of the stochastic complexity can be approximated with the Central Limit Theorem Ziv, These will be used later for computing the control limits either empirically or by methods explained in Ben-Gal et al.
The density of the stochastic complexity is typically a-symmetric, so a correction may be needed for the control bounds. Insert a point in the control charts and monitor it with respect to the UCL and LCL. Machine M2 attempts to process a part whenever it is not starved, i. Machine M1 attempts to process a part whenever it is not blocked. Monitoring the buffer size at regular intervals results in a discrete sequence of buffer states.
For illustration purposes we simulated the queues' state machine to generate a sequence of length 1, Figure 1. The root node presents the system steady-state probabilities and the leaves presents the transition probabilities given the current state: 0. The tree is used for simulating the "out of control" sequences.
As may be well-recognized, the tree shows the probability differences. Note that in this case the presented distributions in both trees, Ta and Tb, can be computed analytically by the first-order Markov process in Figure 1. For validation purposes, we also estimated the parameters of the context tree from a sequence of observations generated by the modified process, which turned out to be accurate within two decimal digits to those represented in Tb. The control limits were determined to be the upper and lower 1.
The stochastic complexity of the lower control limits was found to be 3. Note the doubling the sequence length roughly doubles its mean stochastic complexity. The statistics from 50, monitored sequences of the out-of-control process resulted in detecting Figure 4 presents a typical example for monitoring the stochastic complexity of sequence of length The process is "in control" for the first 50 monitored sequences and deviates of control via the process described by Figure 3 starting from monitored sequence In comparison and as demonstrated in Ben-Gal et al.
Figure 4: A SPC for the stochastic complexity of sequences of length 16; the process changed at the 51th sequence 5. The stochastic complexity measures rely on a context tree as a universal model that can capture complex dependencies in the data, statistical process control in forex. In the given example, statistical process control in forex, the stochastic complexity measure turned out to be sensitive to process changes.
The advantages of the statistical process control in forex method are two-fold: i it is generic and suitable for many types of discrete processes with complex and unknown dependencies; ii it is suitable for relatively short data sequences due to the context tree's convergence properties, statistical process control in forex. The viability statistical process control in forex the statistical process control in forex SPC method and its advantages were illustrated by a numerical experiment.
and Soofi, E. and Shmilovici, A. Related Papers CSPC: A monitoring procedure for state dependent processes By Armin Shmilovici. Context-Based Statistical Process Control By Armin Shmilovici. Measuring the Efficiency of the Intraday Forex Market with a Universal Data Compression Algorithm By Shmuel Hauser.
Using a compressibility measure to distinguish coding and noncoding DNA By Armin Shmilovici. Download pdf. About Press Blog People Papers Job Board We're Hiring! Help Center Find new research papers in: Physics Chemistry Biology Health Sciences Ecology Earth Sciences Cognitive Science Mathematics Computer Science Terms Privacy Copyright Academia ©
Statistical Process Control (SPC) \u0026 it's Importance
, time: 4:02Statistical Process Control: A Guide for Implementation | Emerald Insight
Statistical quality control (SQC) is defined as the application of the 14 statistical and analytical tools (7-QC and 7-SUPP) to monitor process outputs (dependent variables). Statistical process control (SPC) is the application of the same 14 tools to control process inputs (independent variables). Although both terms are often used interchangeably, SQC includes acceptance sampling where SPC does not PPT – Statistical Process Control PowerPoint presentation | free to view - id: 94fff-ZmU4N. The Adobe Flash plugin is needed to view this content. Get the plugin now. Actions. Remove this presentation Flag as Inappropriate I Don't Like This I like this Remember as a Favorite. Share Share 01/04/ · Focuses on the Statistical Process Control (SPC) implementation phase in an effort to underline that SPC is not just control charts, and that many steps have to be accomplished before these charts are used. In addition, highlights the role of training and presents it as Cited by: 25
No comments:
Post a Comment